目錄
- 一、概述
- 二、加噪
- 2.1、第一種:控制噪聲因子
- 2.2、第二種:控制信噪比
- 三、加混響
- 3.1、方法一:Pyroomacoustics實(shí)現(xiàn)音頻加混響
- 3.2、方法二:Image Source Method 算法講解
- 四、生成指定SER的混響
- 五、波形位移
- 六、波形拉伸
- 七、音高修正(Pitch Shifting)
一、概述
音頻時(shí)域波形具有以下特征:音調(diào),響度,質(zhì)量。我們?cè)谶M(jìn)行數(shù)據(jù)增強(qiáng)時(shí),最好只做一些小改動(dòng),使得增強(qiáng)數(shù)據(jù)和源數(shù)據(jù)存在較小差異即可,切記不能改變?cè)袛?shù)據(jù)的結(jié)構(gòu),不然將產(chǎn)生“臟數(shù)據(jù)”,通過對(duì)音頻數(shù)據(jù)進(jìn)行數(shù)據(jù)增強(qiáng),能有助于我們的模型避免過度擬合并變得更加通用。
我發(fā)現(xiàn)對(duì)聲波的以下改變是有用的:Noise addition(增加噪音)、Add reverb(增加混響)、Time shifting(時(shí)移)、Pitch shifting(改變音調(diào))和Time stretching(時(shí)間拉伸)。
本章需要使用的python庫(kù):
- matplotlib:繪制圖像
- librosa:音頻數(shù)據(jù)處理
- numpy:矩陣數(shù)據(jù)處理
使用先畫出原始語音數(shù)據(jù)的語譜圖和波形圖
import librosa
import numpy as np
import matplotlib.pyplot as plt
plt.rcParams['font.sans-serif'] = ['SimHei'] # 用來正常顯示中文標(biāo)簽
plt.rcParams['axes.unicode_minus'] = False # 用來正常顯示符號(hào)
fs = 16000
wav_data, _ = librosa.load("./p225_001.wav", sr=fs, mono=True)
# ########### 畫圖
plt.subplot(2, 2, 1)
plt.title("語譜圖", fontsize=15)
plt.specgram(wav_data, Fs=16000, scale_by_freq=True, sides='default', cmap="jet")
plt.xlabel('秒/s', fontsize=15)
plt.ylabel('頻率/Hz', fontsize=15)
plt.subplot(2, 2, 2)
plt.title("波形圖", fontsize=15)
time = np.arange(0, len(wav_data)) * (1.0 / fs)
plt.plot(time, wav_data)
plt.xlabel('秒/s', fontsize=15)
plt.ylabel('振幅', fontsize=15)
plt.tight_layout()
plt.show()

二、加噪
添加的噪聲為均值為0,標(biāo)準(zhǔn)差為1的高斯白噪聲,有兩種方法對(duì)數(shù)據(jù)進(jìn)行加噪
2.1、第一種:控制噪聲因子
def add_noise1(x, w=0.004):
# w:噪聲因子
output = x + w * np.random.normal(loc=0, scale=1, size=len(x))
return output
Augmentation = add_noise1(x=wav_data, w=0.004)

2.2、第二種:控制信噪比
通過信噪比的公式推導(dǎo)出噪聲。
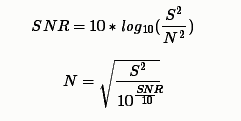
def add_noise2(x, snr):
# snr:生成的語音信噪比
P_signal = np.sum(abs(x) ** 2) / len(x) # 信號(hào)功率
P_noise = P_signal / 10 ** (snr / 10.0) # 噪聲功率
return x + np.random.randn(len(x)) * np.sqrt(P_noise)
Augmentation = add_noise2(x=wav_data, snr=50)
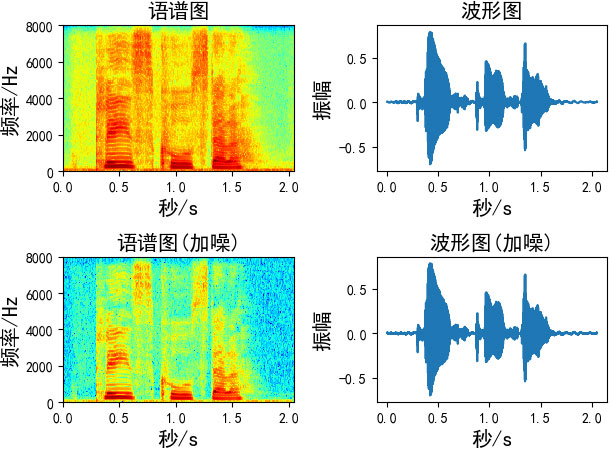
三、加混響
我這里使用的是Image Source Method(鏡像源方法)來實(shí)現(xiàn)語音加混響,我想用兩種方法來給大家實(shí)現(xiàn),第一種是直接調(diào)用python庫(kù)——Pyroomacoustics來實(shí)現(xiàn)音頻加混響,第二種就是按照公式推導(dǎo)一步一步來實(shí)現(xiàn),兩種效果一樣,想看細(xì)節(jié)的可以參考第二種方法,只想開始實(shí)現(xiàn)效果的可以只看第一種方法:
3.1、方法一:Pyroomacoustics實(shí)現(xiàn)音頻加混響
首先需要安裝Pyroomacoustics,這個(gè)庫(kù)非常強(qiáng)大,感興趣也可以多看看其他API接口
pip install Pyroomacoustics
步驟:
1.創(chuàng)建房間(定義房間大小、所需的混響時(shí)間、墻面材料、允許的最大反射次數(shù)、)
2.在房間內(nèi)創(chuàng)建信號(hào)源
3.在房間內(nèi)放置麥克風(fēng)
4.創(chuàng)建房間沖擊響應(yīng)
5.模擬聲音傳播
# -*- coding:utf-8 -*-
import pyroomacoustics as pra
import numpy as np
import matplotlib.pyplot as plt
import librosa
# 1、創(chuàng)建房間
# 所需的混響時(shí)間和房間的尺寸
rt60_tgt = 0.5 # 所需的混響時(shí)間,秒
room_dim = [9, 7.5, 3.5] # 我們定義了一個(gè)9m x 7.5m x 3.5m的房間,米
# 我們可以使用Sabine's公式來計(jì)算壁面能量吸收和達(dá)到預(yù)期混響時(shí)間所需的ISM的最大階數(shù)(RT60,即RIR衰減60分貝所需的時(shí)間)
e_absorption, max_order = pra.inverse_sabine(rt60_tgt, room_dim) # 返回 墻壁吸收的能量 和 允許的反射次數(shù)
# 我們還可以自定義 墻壁材料 和 最大反射次數(shù)
# m = pra.Material(energy_absorption="hard_surface") # 定義 墻的材料,我們還可以定義不同墻面的的材料
# max_order = 3
room = pra.ShoeBox(room_dim, fs=16000, materials=pra.Material(e_absorption), max_order=max_order)
# 在房間內(nèi)創(chuàng)建一個(gè)位于[2.5,3.73,1.76]的源,從0.3秒開始向仿真中發(fā)出wav文件的內(nèi)容
audio, _ = librosa.load("speech.wav",sr=16000) # 導(dǎo)入一個(gè)單通道語音作為源信號(hào) source signal
room.add_source([2.5, 3.73, 1.76], signal=audio, delay=0.3)
# 3、在房間放置麥克風(fēng)
# 定義麥克風(fēng)的位置:(ndim, nmics) 即每個(gè)列包含一個(gè)麥克風(fēng)的坐標(biāo)
# 在這里我們創(chuàng)建一個(gè)帶有兩個(gè)麥克風(fēng)的數(shù)組,
# 分別位于[6.3,4.87,1.2]和[6.3,4.93,1.2]。
mic_locs = np.c_[
[6.3, 4.87, 1.2], # mic 1
[6.3, 4.93, 1.2], # mic 2
]
room.add_microphone_array(mic_locs) # 最后將麥克風(fēng)陣列放在房間里
# 4、創(chuàng)建房間沖擊響應(yīng)(Room Impulse Response)
room.compute_rir()
# 5、模擬聲音傳播,每個(gè)源的信號(hào)將與相應(yīng)的房間脈沖響應(yīng)進(jìn)行卷積。卷積的輸出將在麥克風(fēng)上求和。
room.simulate()
# 保存所有的信號(hào)到wav文件
room.mic_array.to_wav("./guitar_16k_reverb_ISM.wav", norm=True, bitdepth=np.float32,)
# 測(cè)量混響時(shí)間
rt60 = room.measure_rt60()
print("The desired RT60 was {}".format(rt60_tgt))
print("The measured RT60 is {}".format(rt60[1, 0]))
plt.figure()
# 繪制其中一個(gè)RIR. both can also be plotted using room.plot_rir()
rir_1_0 = room.rir[1][0] # 畫出 mic 1和 source 0 之間的 RIR
plt.subplot(2, 1, 1)
plt.plot(np.arange(len(rir_1_0)) / room.fs, rir_1_0)
plt.title("The RIR from source 0 to mic 1")
plt.xlabel("Time [s]")
# 繪制 microphone 1 處接收到的信號(hào)
plt.subplot(2, 1, 2)
plt.plot(np.arange(len(room.mic_array.signals[1, :])) / room.fs, room.mic_array.signals[1, :])
plt.title("Microphone 1 signal")
plt.xlabel("Time [s]")
plt.tight_layout()
plt.show()
room = pra.ShoeBox(
room_dim,
fs=16000,
materials=pra.Material(e_absorption),
max_order=3,
ray_tracing=True,
air_absorption=True,
)
# 激活射線追蹤
room.set_ray_tracing()
room.simulate(reference_mic=0, snr=10) # 控制信噪比


3.2、方法二:Image Source Method 算法講解
從這里要講算法和原理了,
代碼參考:matlab版本:RIR-Generator,python版本:rir-generator
鏡像源法簡(jiǎn)介:

將反射面等效為一個(gè)虛像,或者說鏡像。比如說,在一個(gè)開放空間里有一面平整墻面,那么一個(gè)聲源可以等效為2兩個(gè)聲源;一個(gè)開放空間里有兩面垂直的平整墻面,那么一個(gè)聲源可以等效為4個(gè);同理三面的話是8個(gè)。原理上就是這樣,但是封閉的三維空間里情況有那么點(diǎn)復(fù)雜,
一般來說,家里的空房間可以一定程度上近似為矩形盒子,假設(shè)房間尺寸為:
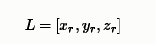
元素大小分別代表長(zhǎng)寬高,而聲源的三維坐標(biāo)為
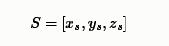
麥克風(fēng)的三維坐標(biāo)為
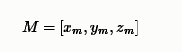
鏡像聲源$(i,j,k)$到麥克風(fēng)距離在三個(gè)坐標(biāo)軸上的位置為
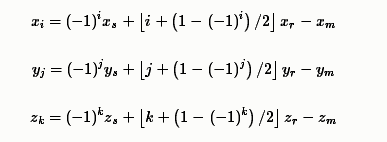
那么聲源$(i,j,k)$距離麥克風(fēng)的距離為
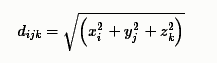
相對(duì)于直達(dá)聲的到達(dá)延遲時(shí)間為
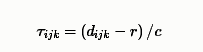
其中$c$為聲速,$r$為聲源到麥克風(fēng)的直線距離。那么,混響效果等效為不同延遲的信號(hào)的疊加,即混響效果可以表示為一個(gè)FIR濾波器與信號(hào)源卷積的形式,此濾波器可寫為如下形式
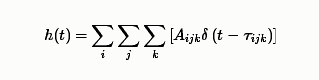
濾波器的抽頭系數(shù)與鏡面的反射系數(shù)與距離相關(guān),如果每個(gè)面的反射系數(shù)不同則形式略復(fù)雜。詳細(xì)代碼還是要看RIR-Generator,我這里只做拋轉(zhuǎn)引玉,寫一個(gè)最簡(jiǎn)單的。
模擬鏡像源:
房間尺寸(m):4 X 4 X 3
聲源坐標(biāo)(m):2 X 2 X 0
麥克風(fēng)坐標(biāo)(m):2 X 2 X 1.5
混響時(shí)間(s):0.2
RIR長(zhǎng)度:512
clc;clear;
c = 340; % 聲速 (m/s)
fs = 16000; % Sample frequency (samples/s)
r = [2 2 1.5]; % 麥克風(fēng)位置 [x y z] (m)
s = [2 2 0]; % 揚(yáng)聲器位置 [x y z] (m)
L = [4 4 3]; % 房間大小 [x y z] (m)
beta = 0.2; % 混響時(shí)間 (s)
n = 512; % RIR長(zhǎng)度
h = rir_generator(c, fs, r, s, L, beta, n);
disp(size(h)) % (1,4096)
[speech, fs] = audioread("./test_wav/p225_001.wav");
disp(size(speech)); % (46797,1)
y = conv(speech', h);
disp(length(y))
% 開始畫圖
figure('color','w'); % 背景色設(shè)置成白色
subplot(3,1,1)
plot(h)
title("房間沖擊響應(yīng) RIR","FontSize",14)
subplot(3,2,3)
plot(speech)
title("原語音波形","FontSize",14)
subplot(3,2,4)
plot(y)
title("加混響語音波形","FontSize",14)
subplot(3,2,5)
specgram(speech,512,fs,512,256);
title("原語音頻譜","FontSize",14)
subplot(3,2,6)
specgram(y,512,fs,512,256);
title("加混響語音頻譜","FontSize",14)
audiowrite("./test_wav/matlab_p225_001_reverber.wav",y,fs)

四、生成指定SER的混響
SER的公式為
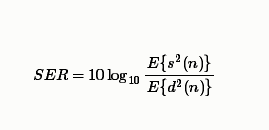
其中E是統(tǒng)計(jì) 期望操作,$s(n)$是近端語音,$d(n)$是遠(yuǎn)端回聲,
由于我們需要根據(jù)指定的SER求混響信號(hào),并且近端語音和遠(yuǎn)端混響都是已知的,我們只需要求得一個(gè)系數(shù),來調(diào)整回聲信號(hào)的能量大小,與遠(yuǎn)端混響相乘即可得我們想要的混響語音,即調(diào)整后的回聲信號(hào)為$kd(n)$
根據(jù)以上公式,可以推導(dǎo)出$k$的值
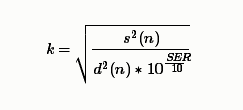
最終$kd(n)$即我們所求的指定SER的混響。
def add_echo_ser(near_speech, far_echo, SER):
"""根據(jù)指定的SER求回聲
:param near_speech: 近端語音
:param far_echo: 遠(yuǎn)端回聲
:param SER: 指定的SER
:return: 指定SER的回聲
"""
p_near_speech = np.mean(near_speech ** 2) # 近端語音功率
p_far_echo = np.mean(far_echo ** 2) # 遠(yuǎn)端回聲功率
k = np.sqrt(p_near_speech / (10 ** (SER / 10)) / p_far_echo)
return k * far_echo

五、波形位移
語音波形移動(dòng)使用numpy.roll函數(shù)向右移動(dòng)shift距離
numpy.roll(a,shift,axis=None)
參數(shù):
- a:數(shù)組
- shift:滾動(dòng)的長(zhǎng)度
- axis:滾動(dòng)的維度。0為垂直滾動(dòng),1為水平滾動(dòng),參數(shù)為None時(shí),會(huì)先將數(shù)組扁平化,進(jìn)行滾動(dòng)操作后,恢復(fù)原始形狀
x = np.arange(10)
# array([0, 1, 2, 3, 4, 5, 6, 7, 8, 9])
print(np.roll(x, 2))
# array([8, 9, 0, 1, 2, 3, 4, 5, 6, 7])
波形位移函數(shù):
def time_shift(x, shift):
# shift:移動(dòng)的長(zhǎng)度
return np.roll(x, int(shift))
Augmentation = time_shift(wav_data, shift=fs//2)

六、波形拉伸
在不影響音高的情況下改變聲音的速度 / 持續(xù)時(shí)間。這可以使用librosa的time_stretch函數(shù)來實(shí)現(xiàn)。
def time_stretch(x, rate):
# rate:拉伸的尺寸,
# rate > 1 加快速度
# rate 1 放慢速度
return librosa.effects.time_stretch(x, rate)
Augmentation = time_stretch(wav_data, rate=2)

七、音高修正(Pitch Shifting)
音高修正只改變音高而不影響音速,我發(fā)現(xiàn)-5到5之間的步數(shù)更合適
def pitch_shifting(x, sr, n_steps, bins_per_octave=12):
# sr: 音頻采樣率
# n_steps: 要移動(dòng)多少步
# bins_per_octave: 每個(gè)八度音階(半音)多少步
return librosa.effects.pitch_shift(x, sr, n_steps, bins_per_octave=bins_per_octave)
# 向上移三音(如果bins_per_octave為12,則六步)
Augmentation = pitch_shifting(wav_data, sr=fs, n_steps=6, bins_per_octave=12)
# 向上移三音(如果bins_per_octave為24,則3步)
Augmentation = pitch_shifting(wav_data, sr=fs, n_steps=3, bins_per_octave=24)
# 向下移三音(如果bins_per_octave為12,則六步)
Augmentation = pitch_shifting(wav_data, sr=fs, n_steps=-6, bins_per_octave=12)

還有寫沒有跑通,但是總感覺有些價(jià)值的代碼,記錄在這里:
py-RIR-Generator(沒跑通的原因是我是window系統(tǒng))gpuRIR(這個(gè)我跑通了,但是需要較大的計(jì)算資源)去github找代碼的時(shí)候,不一定要搜索“回聲”,“混響”,也可以通過搜索"RIR"同樣可以得到想要的結(jié)果
本文畫圖代碼:
# Author:凌逆戰(zhàn)
# -*- coding:utf-8 -*-
import matplotlib.pyplot as plt
import librosa
import numpy as np
plt.rcParams['font.sans-serif']=['SimHei'] #用來正常顯示中文標(biāo)簽
plt.rcParams['axes.unicode_minus']=False #用來正常顯示符號(hào)
y1, _ = librosa.load("./speech.wav", sr=16000)
y2, _ = librosa.load("./guitar_16k_reverb_ISM.wav", sr=16000)
plt.subplot(2, 2, 1)
plt.specgram(y1, Fs=16000, scale_by_freq=True, sides='default', cmap="jet")
plt.title("語譜圖", fontsize=13)
plt.xlabel('時(shí)間/s', fontsize=13)
plt.ylabel('頻率/Hz', fontsize=13)
plt.subplot(2, 2, 2)
plt.plot(np.arange(len(y1)) / 16000, y1)
plt.title("波形圖", fontsize=13)
plt.xlabel('時(shí)間/s', fontsize=13)
plt.ylabel('振幅', fontsize=13)
plt.subplot(2, 2, 3)
plt.specgram(y2, Fs=16000, scale_by_freq=True, sides='default', cmap="jet")
plt.title("語譜圖(加混響)", fontsize=13)
plt.xlabel('時(shí)間/s', fontsize=13)
plt.ylabel('頻率/Hz', fontsize=13)
plt.subplot(2, 2, 4)
plt.plot(np.arange(len(y2)) / 16000, y2)
plt.title("波形圖(加混響)", fontsize=13)
plt.xlabel('時(shí)間/s', fontsize=13)
plt.ylabel('振幅', fontsize=13)
plt.tight_layout()
plt.show()
以上就是分析語音數(shù)據(jù)增強(qiáng)及python實(shí)現(xiàn)的詳細(xì)內(nèi)容,更多關(guān)于語音數(shù)據(jù)增強(qiáng) python實(shí)現(xiàn)的資料請(qǐng)關(guān)注腳本之家其它相關(guān)文章!
您可能感興趣的文章:- python3實(shí)現(xiàn)語音轉(zhuǎn)文字(語音識(shí)別)和文字轉(zhuǎn)語音(語音合成)
- python語音識(shí)別指南終極版(有這一篇足矣)
- 使用Python和百度語音識(shí)別生成視頻字幕的實(shí)現(xiàn)
- 基于python實(shí)現(xiàn)語音錄入識(shí)別代碼實(shí)例
- Python譜減法語音降噪實(shí)例
- python實(shí)現(xiàn)智能語音天氣預(yù)報(bào)
- Python(PyS60)實(shí)現(xiàn)簡(jiǎn)單語音整點(diǎn)報(bào)時(shí)
- python文字轉(zhuǎn)語音實(shí)現(xiàn)過程解析
- 使用python實(shí)現(xiàn)語音文件的特征提取方法